AI-based insurance rate calculator
Every policyholder has their own individual tariff
- It all began with a successful project at Signal Iduna Versicherung in 2018.
- We have now welcomed the third and fourth largest insurance companies in China and the No. 5 in Japan as customers.
Challenges
In the fascinating world of insurance, specifically travel insurance, HighPots has reached a milestone. Our AI product for travel insurance has changed the way rates are calculated and exceeded the expectations of insurance companies. Previously, travel insurance rates were dependent on rigid statistical calculations where actuaries relied on historical data to make risk assessments. These traditional approaches resulted in standard rates that often failed to keep pace with the dynamic reality of the travel market. We started the project in 2018 with Signal Iduna in the area of travel insurance (travel cancellation and travel health insurance). The following challenges had to be overcome:
The following challenges had to be overcome:
Procurement of travel data
Validation of the data
Structuring and parsing the data
Relevance of the different travel dates
Testing whether the AI system is better than that of the actuaries
Automated return and evaluation of cancellations and claims
Procedure
Some of the HighPots ML components changed this back in 2018 for a German insurance group. The system has since become more intelligent and comprehensive.
In real time, the AI analyzes significantly more parameters than just the classics, such as trip duration, age of the travelers, travel price, vacation destination or the travelers’ place of residence. Each individual traveler now receives a tailor-made insurance tariff that precisely reflects their individual risks and needs. In the end, this not only ensures fair treatment of travelers, but also financial benefits for the insurance companies.
However, the HighPots Insurance AI goes far beyond simply calculating rates – it is constantly improving. Through continuous learning, not only from claims that have occurred but also from non-occurring scenarios, the AI constantly improves its forecasting capabilities in real time. The result is a precise risk classification in real time that offers insurance companies an unprecedented level of risk calculation.
Results
And this is just the beginning. In the next version of the AI product, we will not only be working on perfecting individual insurance rates, but also on designing individual contract terms that match the individual insurance rates. This will not only make insurance pricing more personalized, but also more transparent and flexible. AI will also be extended to a neighboring area of insurance, namely health insurance. The difference between travel health insurance and health insurance is not as great as one might think at first glance.
The AI was trained using data provided to us by GDS providers such as Amadeus and data from insurance companies. The HighPots AI is currently being tested at a Japanese insurance company.
The initial results are very promising. The contact person for this product is our Head of Research and Development, Rüdiger Off (ruediger.off@highpots.com).
Travel insurance - profits for insurance companies and consumers before and after the use of AI
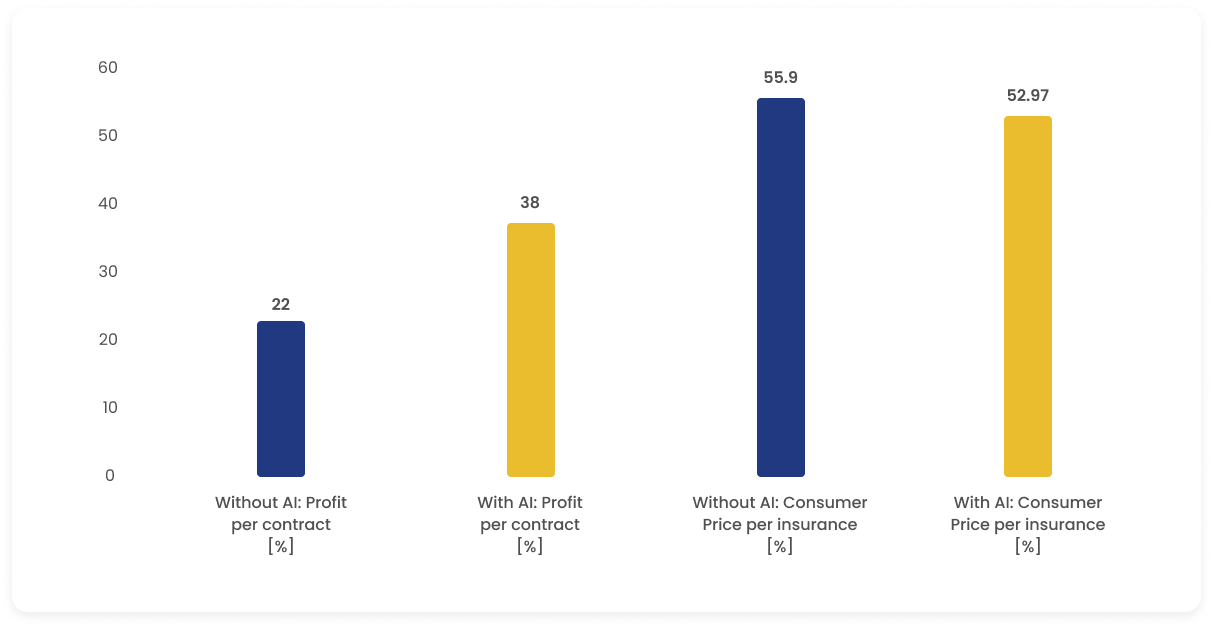
Analyzed number
100 000
Trip type
Package tour
Age of travelers
Between 40 and 60 years
Type of insurance
Travel insurance per vacation
Number of insurance companies
5 different insurance companies
AI environments
TensorFlow with permanent learning in real time (HighPots AI Open Source Cloud).
Surprising for insurance groups
Age is a decisive parameter, but that does not mean that the oldest people are the biggest cost drivers.
Insurance rates
Comparable insurance benefits in the areas of travel health and travel cancellation insurance with all 5 insurance companies.
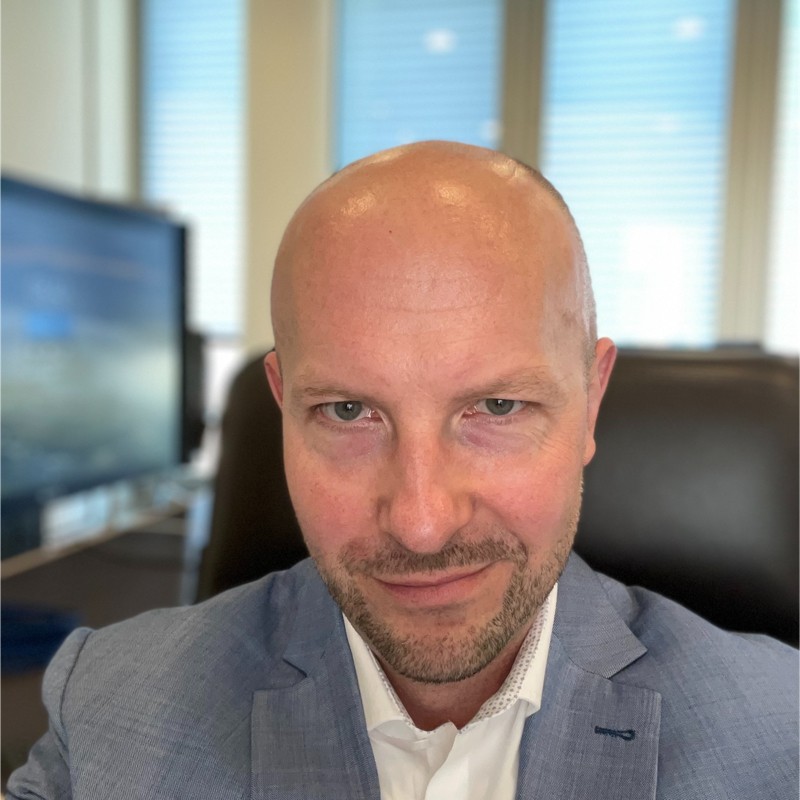
Michael Berlin
Conclusion
- We now have proof that actuarial mathematics is similar to meteorology: statistical statements from well-trained expert systems (AI) lead to a better risk assessment and therefore to greater financial returns for insurance companies than conventional actuarial mathematics. It is important that the AI can permanently participate in and learn from real-time sales and claims, i.e. the real insurance business. The generation of manual intervention options makes sense not only from the point of view of legislation, even if they may never be used. To this end, the establishment of automated threshold values that enable alerts and the associated manual intervention must be implemented.